Synthetic Data Is a Dangerous Teacher
Synthetic Data Is a Dangerous Teacher
Synthetic data, or data that is artificially generated rather than collected from real-world sources, is becoming increasingly...
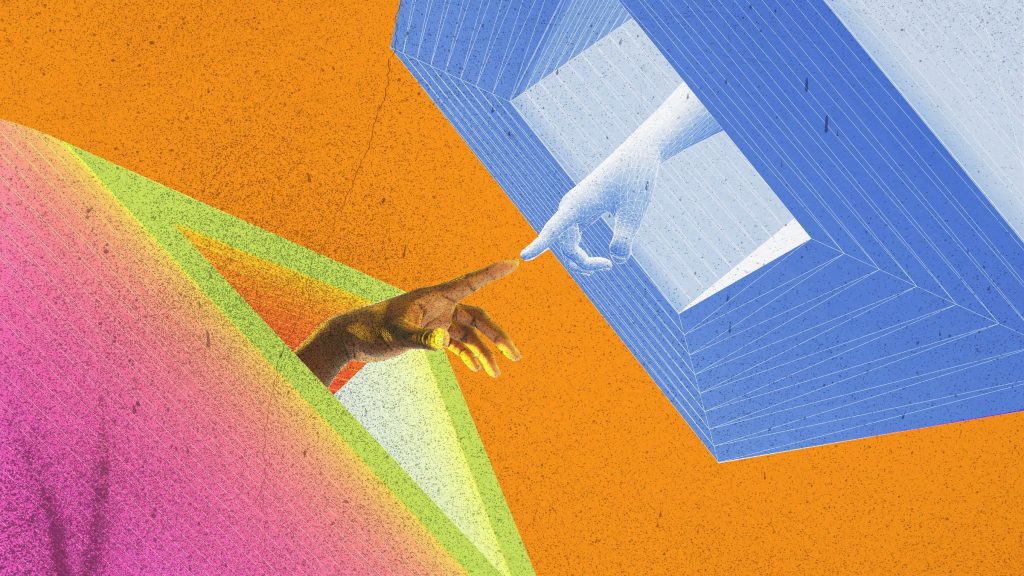
Synthetic Data Is a Dangerous Teacher
Synthetic data, or data that is artificially generated rather than collected from real-world sources, is becoming increasingly popular in the field of machine learning and artificial intelligence. While synthetic data can be useful for training algorithms and creating realistic simulations, it also comes with its own set of dangers.
One of the main risks of relying on synthetic data is that it may not accurately represent the complexities and nuances of real-world data. Algorithms trained on synthetic data may not perform well when applied to actual, messy, and unpredictable data sets.
Furthermore, relying too heavily on synthetic data can lead to overfitting, where algorithms perform well on the training data but fail to generalize to new, unseen data. This can result in serious consequences, especially in high-stakes applications such as healthcare or finance.
Another danger of synthetic data is the potential for bias and discrimination to be amplified in the models trained on it. If the synthetic data is not inclusive and representative of diverse populations, the resulting algorithms may perpetuate existing inequalities and unfair treatment.
In order to mitigate these risks, it is important for researchers and practitioners to carefully consider the limitations of synthetic data and to supplement it with real-world data whenever possible. Additionally, transparency and ethical considerations must be paramount when using synthetic data in algorithm development.
Overall, while synthetic data can be a valuable tool in machine learning, it is essential to approach it with caution and to understand its limitations. Blindly trusting synthetic data as a teacher can lead to disastrous consequences and hinder progress in the field.